Author information
- 1Department of Pediatrics, School of Medicine, College of Medicine, Kaohsiung Medical University Hospital Kaohsiung, Medical University, 100, Shih-Chuan 1st Road, Kaohsiung 80708, Taiwan.2Department of Information Engineering, I-Shou University, No. 1, Section 1, Syuecheng Road, Dashu District, Kaohsiung 84001, Taiwan.3Departments of Electrical Engineering, I-Shou University, No. 1, Section 1, Syuecheng Road, Dashu District, Kaohsiung 84001, Taiwan.4Department of Computer and Communication, National Pingtung University, 51 Min Sheng East Road, Pingtung, 90003, Taiwan.
Abstract
Numerous nonepileptic paroxysmal events, such as syncope and psychogenic nonepileptic seizures, may imitate seizures and impede diagnosis. Misdiagnosis can lead to mistreatment, affecting patients' lives considerably. Electroencephalography is commonly used for diagnosing epilepsy. Although on electroencephalograms (EEGs), epileptiform discharges (ED) specifically indicate epilepsy, only approximately 50% of patients with epilepsy have ED in their first EEG. In this study, we developed a deep convolutional neural network (ConvNet)-based classifier to distinguish EEG between patients with epilepsy without ED and controls. Overall, 25 patients with epilepsy without ED in their EEGs and 25 age-matched patients with Tourette syndrome or syncope were enrolled. Their EEGs were classified using the deep ConvNet. When the EEG data without overlapping were used, the accuracy, sensitivity, and specificity were 65.00%, 48.00%, and 82.00%, respectively. The performance measures improved when the input EEG data were augmented through overlapping. With 95% EEG data overlapping, the accuracy, sensitivity, and specificity increased to 80.00%, 70.00%, and 90.00%, respectively. The proposed method could be regarded as a pilot study to demonstrate a proof of concept of a potential diagnostic value of deep ConvNet in patients with epilepsy without ED. Further studies are needed to assist neurologists in distinguishing nonepileptic paroxysmal events from epilepsy.
KEYWORDS:
EEG; Nonepileptic event; deep convolutional neural network; epilepsy; epileptiform discharge
- PMID:
- 30776988
- DOI:
- 10.1142/S0129065718500600
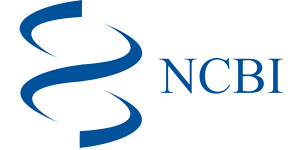